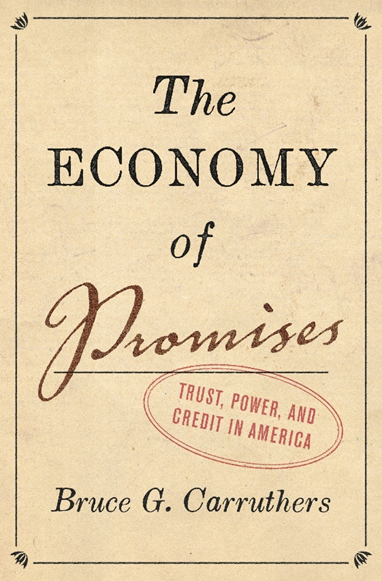
Do you know what a FICO score is, or the value of your own score?
Not everyone does, even though FICO scores are incredibly fateful for individuals and their households. Why? Because today FICO scores govern access to credit, and credit is usually needed to make big purchases (for example, financing a new car, or getting a mortgage to buy a home), to deal with short-term emergency expenses (like surprise medical bills), or to maintain consumption when household income gets interrupted because someone lost their job.
A high score means easier credit, while a low score means expensive credit (higher interest rates) or even no credit at all. That these scores play such a central role should come as no surprise because, in fact, FICO scores were designed to govern access to consumer credit, on a mass scale. But now they are used in other contexts as well, and so have become even more consequential.
Employers use them to evaluate job applicants. Insurance companies incorporate them when they price their products. Landlords use them to decide who to rent to, and they even find applications on dating websites (where a high score presumably makes someone more “attractive”). How did a single number come to shape the life chances of so many people?
Credit ratings: Credit scores for organizations and financial products
If we shift from individuals and consider organizations and corporations instead, it turns out that a similar kind of measure, a bond rating, can be equally significant. Firms that want to borrow money for major capital investments frequently issue long-term bonds, and these are classified by rating agencies into ordered categories, with “AAA” at the top. Non-profits like universities issue bonds and so they too get rated.
Investment banks doing complex securitizations and other kinds of financial engineering pool assets (like home mortgages), and issue asset-backed securities that have to be rated before they can be sold. State and municipal governments also issue bonds to finance their activities, as does the US federal government. In fact, sovereign nations around the world support their public spending by selling bonds.
And, for the most part, a very small group of US-based bond rating agencies (Moody’s, S&P, and Fitch) make fateful decisions by classifying all these bonds as “AAA,” “AA,” or putting them into some other category. Indirectly, these agencies pass judgement on public policy, and almost always reward things like lower taxes and balanced budgets. With a higher rating, the bond issuer can borrow more cheaply whereas lower ratings make everything more expensive.
Why did these ratings come to shape how many organizations and governments obtain resources? Who made bond raters the arbiters of public finance?
Credit ratings as mediators of access to capital
In a financialized economy, access to finance is key, and under capitalism, it matters who can obtain capital. As I argue in my recent book, The Economy of Promises, that access is heavily mediated by numbers, scores, and ratings. These are a social invention created in the mid-19th century in the US to solve a narrow problem, but then expanded, emulated, and repurposed to the point where they have become an integral part of the financial infrastructure.
The initial problem concerned trade credit between businesses: retail stores who ordered goods from their suppliers, and then paid later (say, after 90 days). In effect, the suppliers provided credit to their customers and wanted to be sure they would get paid. Early on, suppliers and customers generally knew each other well: they were part of the same local community or social networks. It was easy to learn whether someone had a reputation for meeting their obligations, or not.
But as commerce expanded mid-century, suppliers increasingly had to deal with strangers who lived far away and who were embedded in different social networks. Whom to trust was a real problem. Private for-profit firms got into the business of collecting credit information, simplifying and translating it into an ordinal category system, and then selling the ratings to clients who wanted to know about the creditworthiness of others. The nationalization of the US economy created enormous demand for succinct, credible information and the new system expanded to encompass the entire country by the end of the century, and went overseas, too.
Even bankers relied on this information when making loans. Such success prompted John Moody to do something similar for railroad bonds, at the start of the 20th-century, and before long he and his competitors were selling their bond ratings to investors, rating corporations, railroads, utilities, and governments. Moody adopted ordinal categories as the canonical format to summarize the complicated financial relationships that determined corporate creditworthiness, and he also sold information to users on a for-profit basis.
Algorithmic quantification of creditworthiness
Today, fintech firms want to take the quantification of creditworthiness to a new level, applying artificial intelligence and machine learning algorithms to petabytes of information in order to surpass incumbent rating agencies in their ability to discern true creditworthiness. Access to credit will be determined not only by a person’s employment history or on-time payments, but also by their social media engagements and web-browsing activity (so be careful who you “friend” on-line!).
Fintech’s “big data” innovations are touted as a way to “democratize” credit and create a more “inclusive” financial system. In light of the well-documented history of racial and gender discrimination in US credit markets, especially when it comes to home mortgages, claims about inclusivity seem particularly compelling. Can algorithms offer a way to avoid bias and increase equity by making lending decisions strictly “by the numbers”?
In reality, they offer no automatic solution to discrimination, and promotional hyperbole needs to be treated with deep skepticism. Trained on data that reflects institutionalized disparities, algorithms could well reproduce patterns of inequality, and their proprietary status protects them from oversight or accountability. Unresolved issues of privacy, data ownership and usage, and cybersecurity remain outstanding as US politicians prove reluctant to constrain a domestic tech sector that has created so much wealth in only a few decades. But the growing possibility of a privately-run digital panopticon makes people nervous.
Leading economic sectors offer the promise of change, and sometimes even for the better. The tech industry has refashioned social life, created new on-line communities, changed politics, transformed commerce, and now proposes to alter our system of credit. Perhaps fintech will move fast and break things like the credit markets that have engendered durable inequalities, building on unprecedented computational capacity to quantify creditworthiness in a granular but neutral way. However, it turns out that mass-quantification wasn’t recently invented by some millennial “tech bro,” but rather started more than 170 years ago. Like the problem of credit inequality, today’s “innovative” solution is actually quite old, and so a historical perspective has much to offer as we confront our challenges and consider a way forward.
Read more
Bruce G. Carruthers. The Economy of Promises: Trust, Power, and Credit in America. Princeton University Press 2023.
No Comments