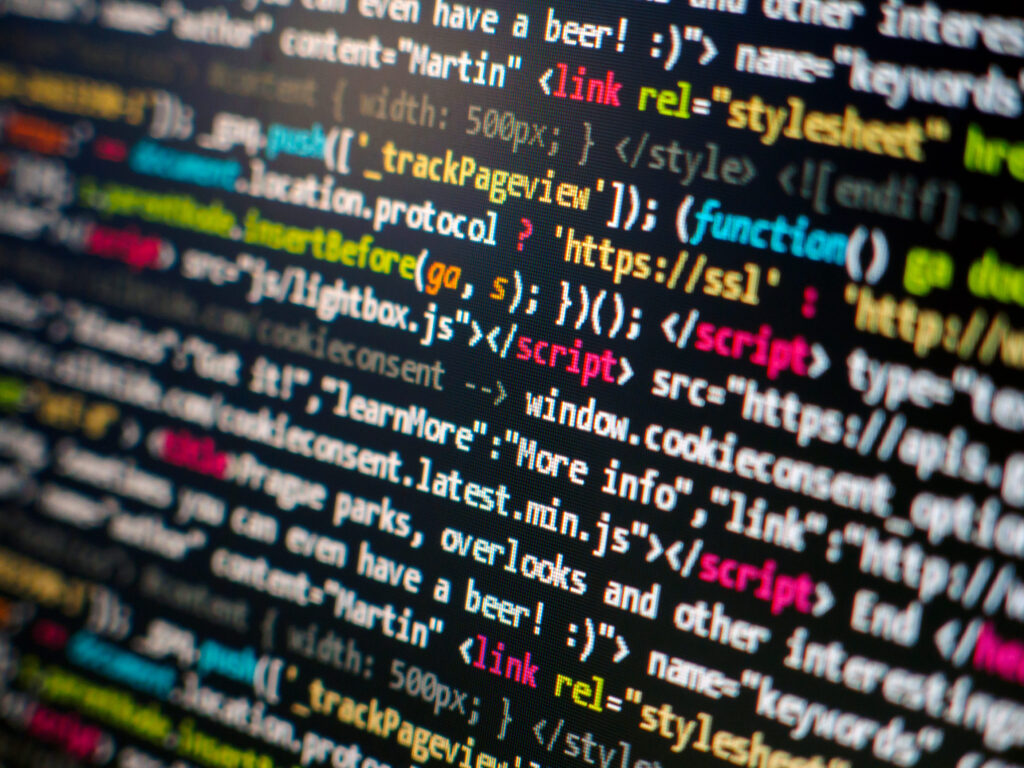
Imagine that you are deciding whether to release a person on bail, grant a consumer a loan, or hire a job candidate. Now imagine your method of making this decision involves using data to algorithmically predict how people will behave—who will skip bail, default on the loan, or be a good employee. How will you know if the way you determine outcomes is fair?
In recent years, computer scientists and others have done a lot to try to answer this question. The flourishing literature on “algorithmic fairness” offers dozens of possibilities, such as testing whether your algorithm predicts equally well for different people, comparing outcomes by race and sex, and assessing how often predictions are incorrect.
Yet something important is missing. There are different ways to evaluate whether benefits and burdens (e.g., loans, tax audits, spots in college) are being allocated fairly. Sometimes, fairness has to do with how one person, or one group of people, is treated relative to another. And sometimes fairness has to do with whether a person gets what they deserve, irrespective of how anyone else is treated.
In a recent article, I argue that the formal aspects of algorithmic prediction and the data it relies on shape our thinking in a way that makes the first type of moral standard more salient than the second. We gravitate to comparative notions of justice while losing sight of noncomparative ones.
To start at the beginning: an algorithm, at its most basic, is a set of rules. Yet today’s algorithms are often data-intensive and computationally complex. A credit scoring algorithm is one example. Credit scores are predictions about how likely a person is to repay a loan. To make this prediction, companies leverage massive databases from credit bureaus. These databases portray people as “cases”—as discrete entities with particular attributes. Picture a spreadsheet with each row representing a person and each column (each of many thousands of columns) representing one small financial detail, like a person’s MasterCard payment from September of last year. Everyone is slotted into the same set of categories, even though the content of those categories may vary. The categories are uniform and exist outside the idiosyncratic context of each person’s life.
This way of rendering people is useful, because it enables the inner workings of algorithms, which look for patterns by comparing people to one another. In the case of credit scoring, the algorithm identifies which patterns of attributes have correlated with people paying loans on-time (or late) in the past, in order to predict who will do so in the future. Portraying people in the standardized way that a spreadsheet does makes comparison easy. But rendering people as “cases” also has its limitations.
To see how, consider another way we might understand people: as actors in the unfolding narratives of their lives. Rather than a spreadsheet, this time the right analogy is a novel. When people are captured in stories, they are dynamic and reactive to the world around them. They exist in a particular social context (the setting) with meaning coming from how things change over time (the plot). Importantly, stories easily reveal how people think and feel. People’s emotions, intentions, and interpretations of events are often what drives the action forward. While cases are good for comparing people to one another, narratives are better at understanding individual people in deep and complex ways.
How does this relate back to fairness? Well, in my article I argue that when we start with people rendered as cases, it’s easier to consider moral standards that rely on comparing one person (or group of people) to another.
For example, if we want to know whether men and women are being treated equally, then it helps to have one set of people flagged as women and another as men—as is simple to do in a spreadsheet. To take another example, if we want to know whether lower and higher income Americans get audited at the same rates, then it is useful to have each person categorized in the same standardized way according to household income.
By contrast, if we want to get at noncomparative justice, narrative is often the more illuminating route. This would be the case in, say, a court, where a judge was figuring out what prison sentence a person deserved. (Moral desert is one type of noncomparative justice.) Here, narrative details of the specific case are often the determinative ones. It’s important to know whether the person who shot the gun knew it was loaded, feared for her life, had tried to talk to her way out of the situation, and so on.
To take a different sort of example, consider the movement to prevent medical debt from being counted in a person’s credit score. Time and again, advocates tell stories about how people can wind up with massive medical debts through no fault of their own. What matters is how a person’s life unfolds, and the role of intentionality (or lack thereof) in events. To hold a person—even just one, single person—to account for something beyond their control would be unfair. Or at least that’s the moral position narrative lets us consider.
Now, none of this is to say that it’s more important to consider one type of fairness or the other. To fully evaluate how algorithms, or any other decision-making tool, allocate, we need to morally reason both comparatively and noncomparatively.
And that means we need to consider people both as cases, in a form that affords comparison, and in narrative, in a form that foregrounds how people interact with their environments in complicated, nuanced, and intentional ways. Those who would dismiss either—cases as antiseptic and heartless or narrative as sentimental and unscientific—are limiting their ability to see the full landscape of morally relevant features.
Read more
Kiviat, Barbara. “The Moral Affordances of Construing People as Cases: How Algorithms and the Data They Depend on Obscure Narrative and Noncomparative Justice” in Sociological Theory 2023.
Kiviat, Barbara. “Which Data Fairly Differentiate? American Views on the Use of Personal Data in Two Market Settings” in Sociological Science 2021.
Kiviat, Barbara. “The Moral Limits of Predictive Practices: The Case of Credit-Based Insurance Scores” in American Sociological Review 2019.
image: Martin Vorel via Wikimedia Commons (CC BY-SA 4.0)
No Comments